Building on Cathedrals
AI-Driven Insights into Architectural Heritage
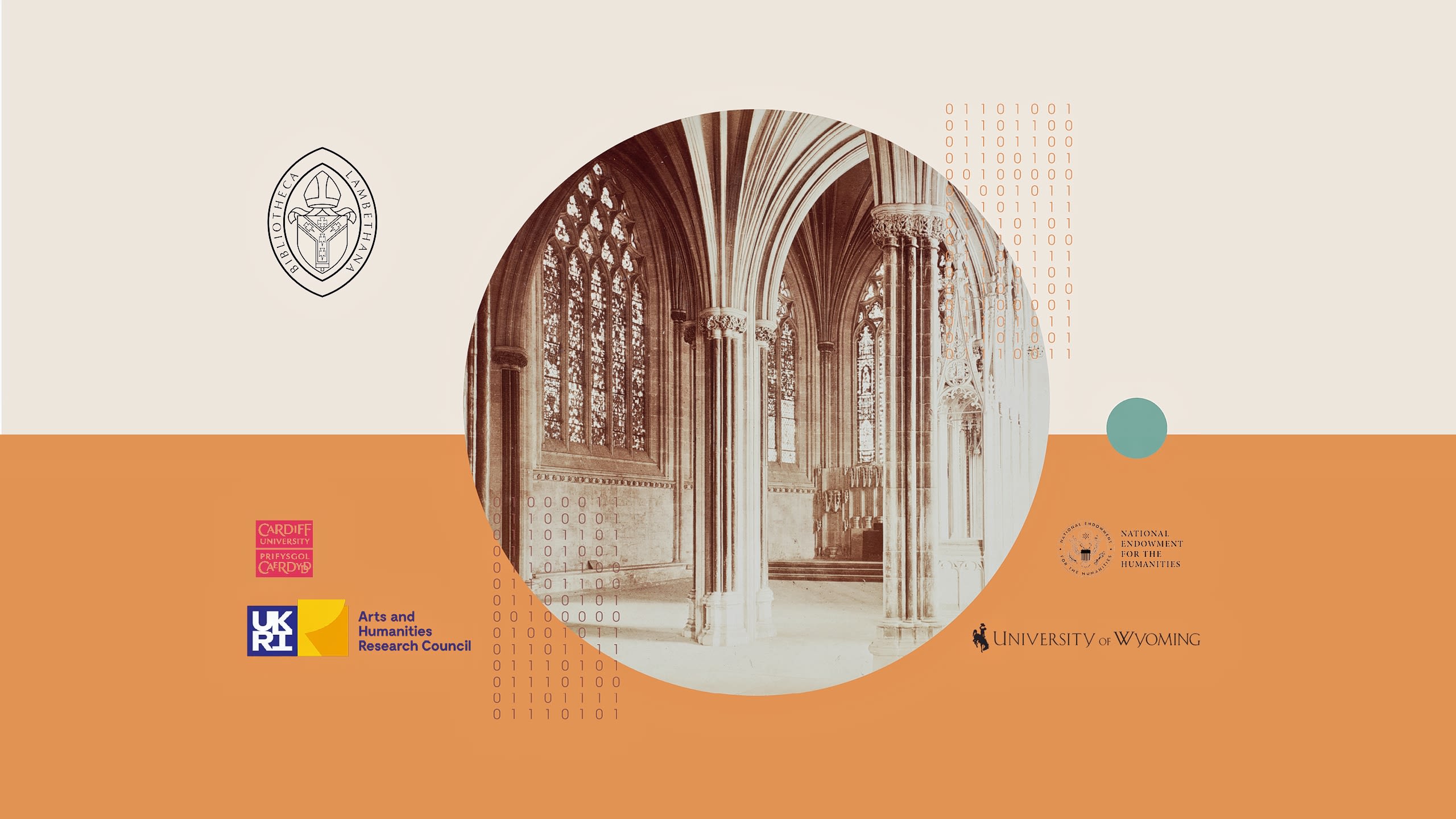
Building on Cathedrals brings together cutting-edge AI technology and Lambeth Palace Library’s rich collections of medieval cathedral architecture. In partnerships with Cardiff University Special Collections and Archives & Wyoming Universities, this project explores how AI-driven tools, such as image recognition, OCR, and caption detection, are enhancing access to England’s ecclesiastical heritage by transforming these historical materials into digital formats.
This digital exhibition, complementing the physical showcase curated and designed by Camille Koutoulakis at Lambeth Palace Library, invites audiences—academics, cultural heritage conservators, architects, and curious minds alike—to explore the intricate world of cathedral architecture. Using innovative technologies, fine-tuned AI classifications, and rich contextual resources, the project and exhibition highlights the intersection of cultural heritage and digital humanities.
Building on Cathedrals is funded by the National Endowment for Humanities [FAIN: HND-284954-22] and the Arts and Humanities Research Council [AH/W005417/1].
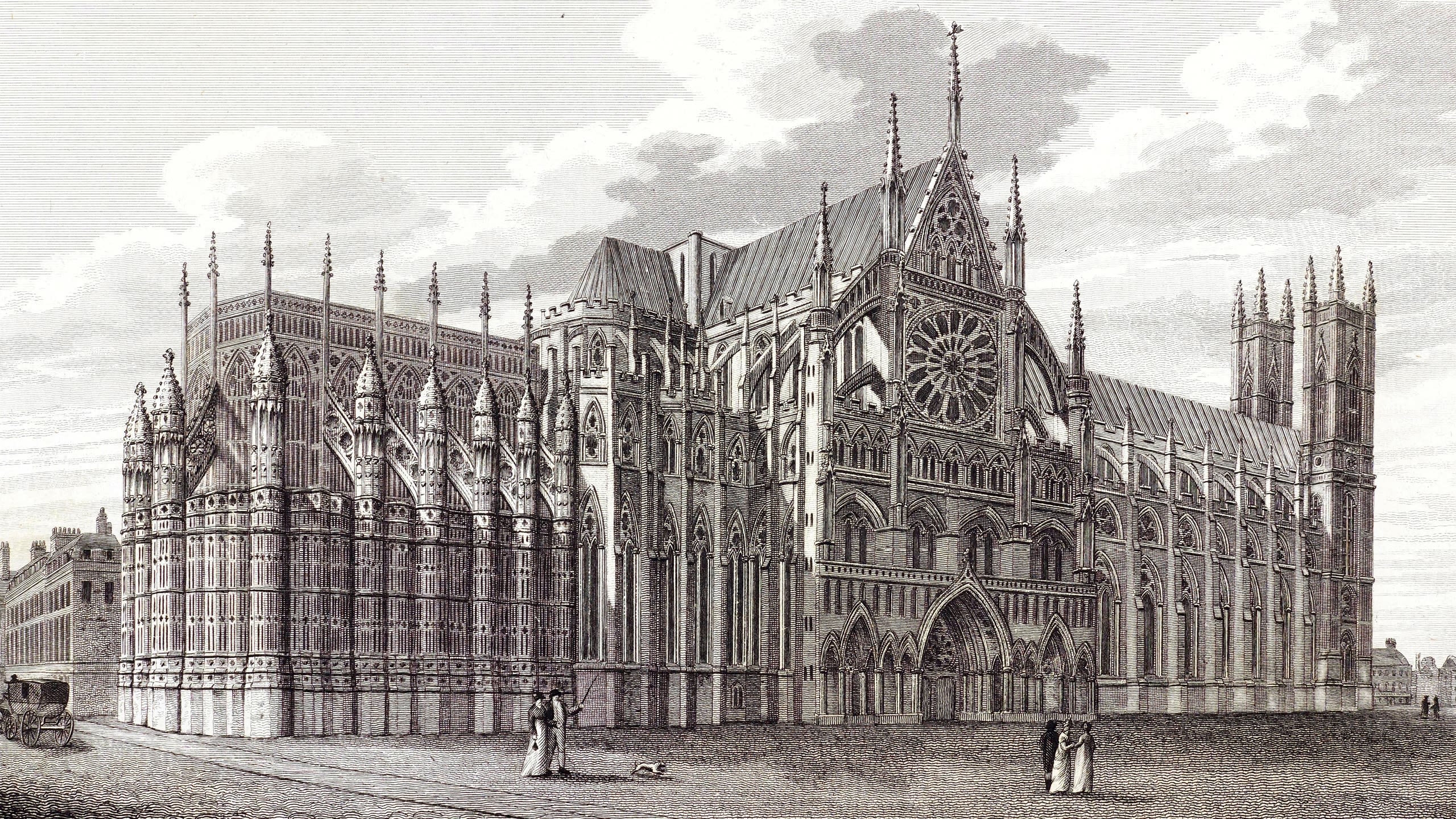
Preserving History in High Definition
With advanced imaging techniques, we’ve photographed over 3,000 images from 22 medieval cathedrals across England, converting delicate historical materials into high-resolution digital formats. This technical endeavour ensures the long-term preservation of invaluable records, while enhancing their accessibility for research and public engagement. By integrating these assets into Lambeth Palace Library’s digital collections, we provide a durable platform for analysis and exploration of medieval architectural heritage.
Unlocking the treasures of our collections
Step into the past with an extraordinary selection of 16th – 19th century prints, including engravings, lithographs, and ground plans. These artworks offer intricate views and technical designs, some by celebrated artists, making them a goldmine for architectural studies. See the full catalogue of images.
Uncover rare 19th – 20th century documentary photographs – like the 19th century glass plates photographs by Thomas Hennell Harrison and Reverend Mann or albumen prints by John W.T. Keene. These photographs provide unparalleled clarity and reveal details of stone carvings, stained glass, and soaring vaults. See the full catalogue of images.
The Cathedrals Fabric Collection of England (CFCE) Cathedral Architecture, Theological and Historical (CATH) collections are brimming with architectural plans, rare prints and fascinating records of cathedral construction and preservation. As the Library team continues to catalogue this material, we are uncovering the stories of these magnificent cathedrals. See the full catalogue of images.
This project isn’t just about preservation; it’s about enhancing access to the study and engagement of medieval cathedral artistry. It explores how AI can reveal details to create new ways of engaging with centuries-old images.
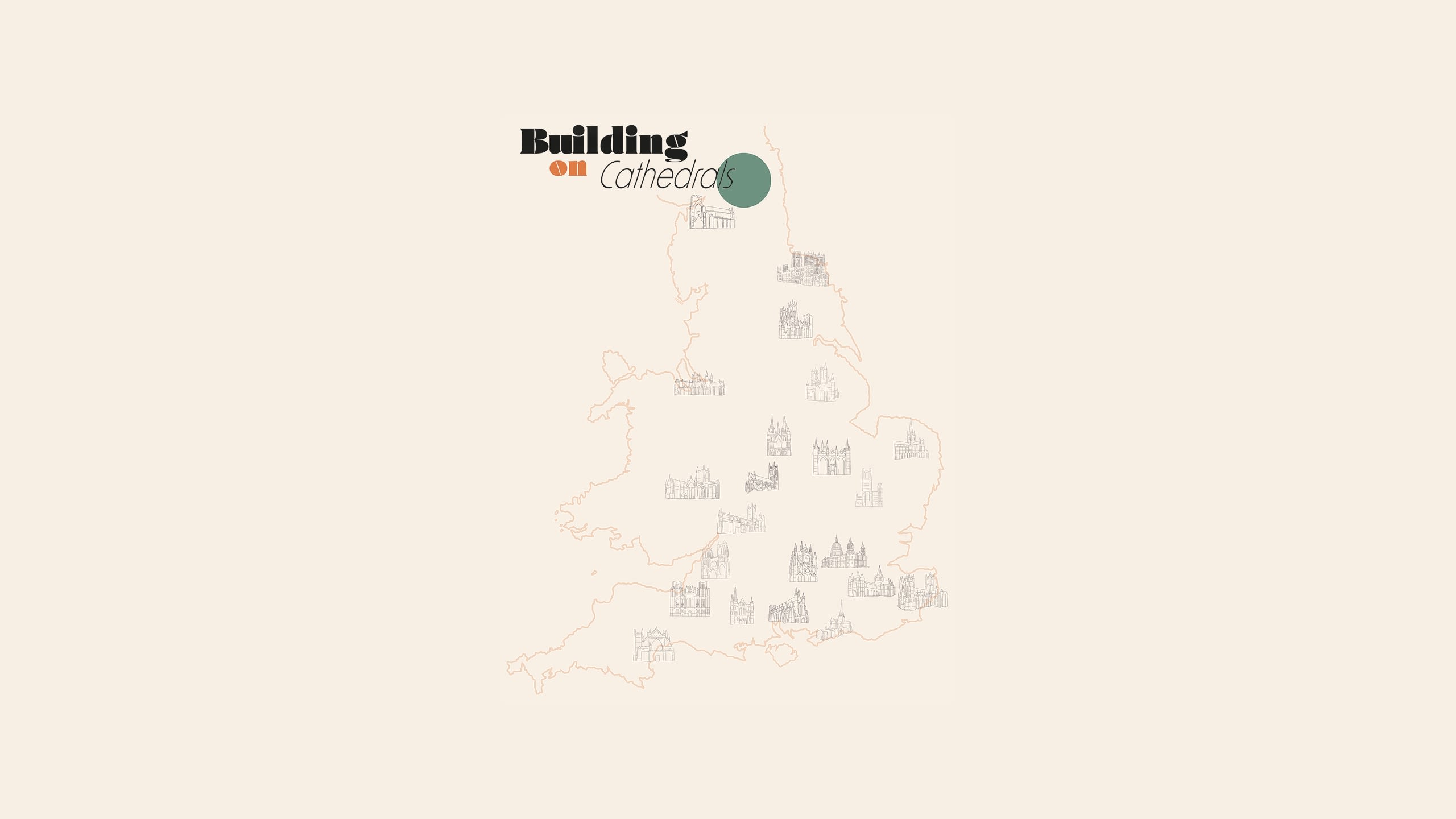
Transforming Cathedral Studies
By employing AI technologies, Lambeth Palace Library can work towards digitising fragile historical materials and make them easily accessible to researchers and the public. These tools also enable the categorisation and analysis of complex architectural features, facilitating deeper insights into the intricate designs of medieval cathedrals.
In total, 1,488 AI-enhanced tags have been added to the collection, further enriching the ability to search, classify, and explore these architectural wonders. This AI-driven classification fosters collaboration among scholars and enhances the library’s role as a dynamic resource for cultural heritage and academic research.
Contrastive Language-Image Pre-Training Model Testing
We used a sample dataset of 2,542 images to evaluate the capabilities of the CLIP (Contrastive Language-Image Pre-Training) model. CLIP leverages Natural Language Processing (NLP) to encode textual information into meaningful vector representations that align with corresponding visual data.
It operates by generating embeddings—vector representations for both images and text—that encapsulate the essential features of the input data.
To begin the analysis, we used CLIP to categorise our dataset into five heading classifications, which helped organise and structure the content. By segmenting the data, we could now delve deeper into each classification enabling more granular analysis and providing insights into architectural features across the collection.
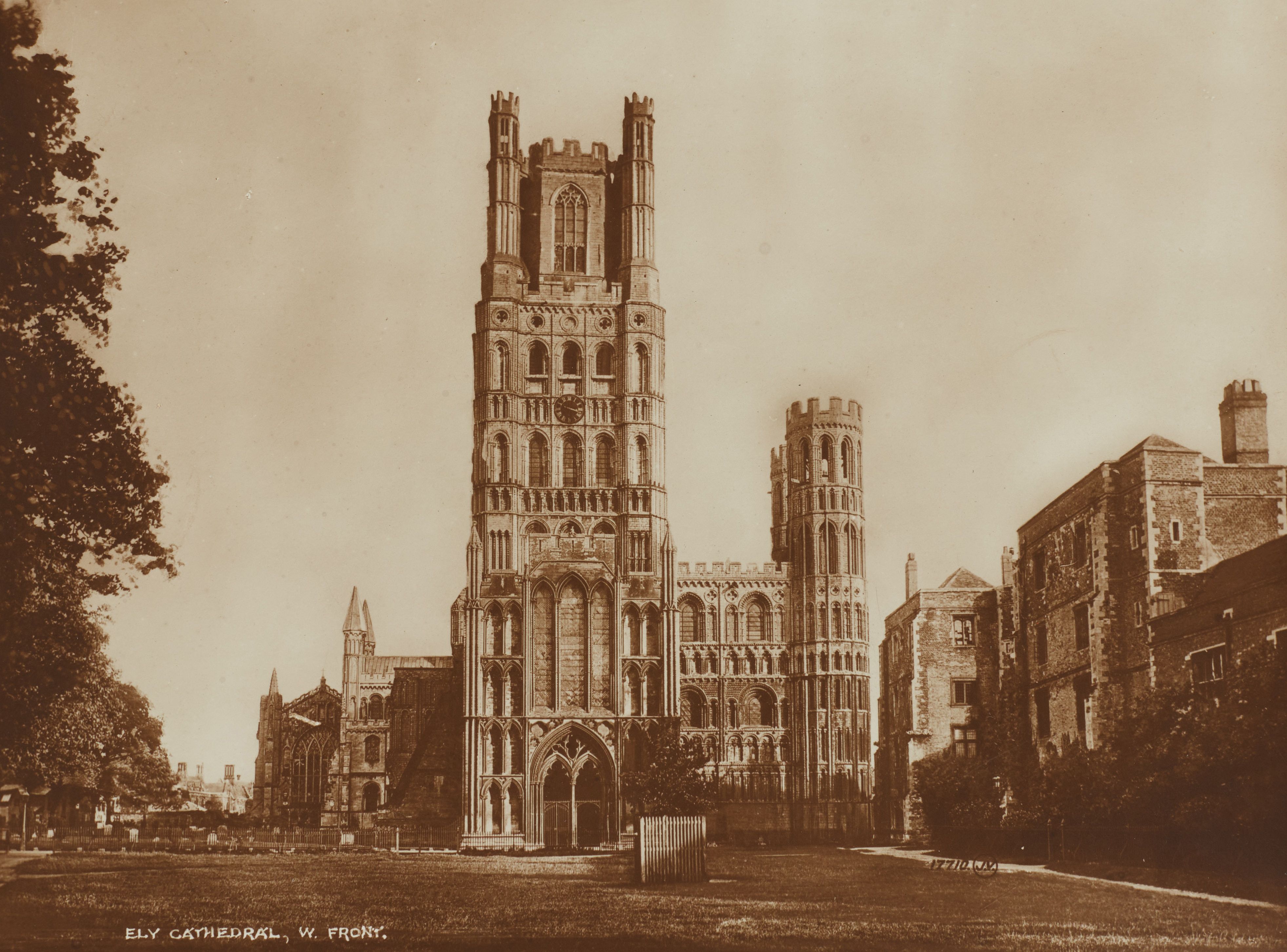
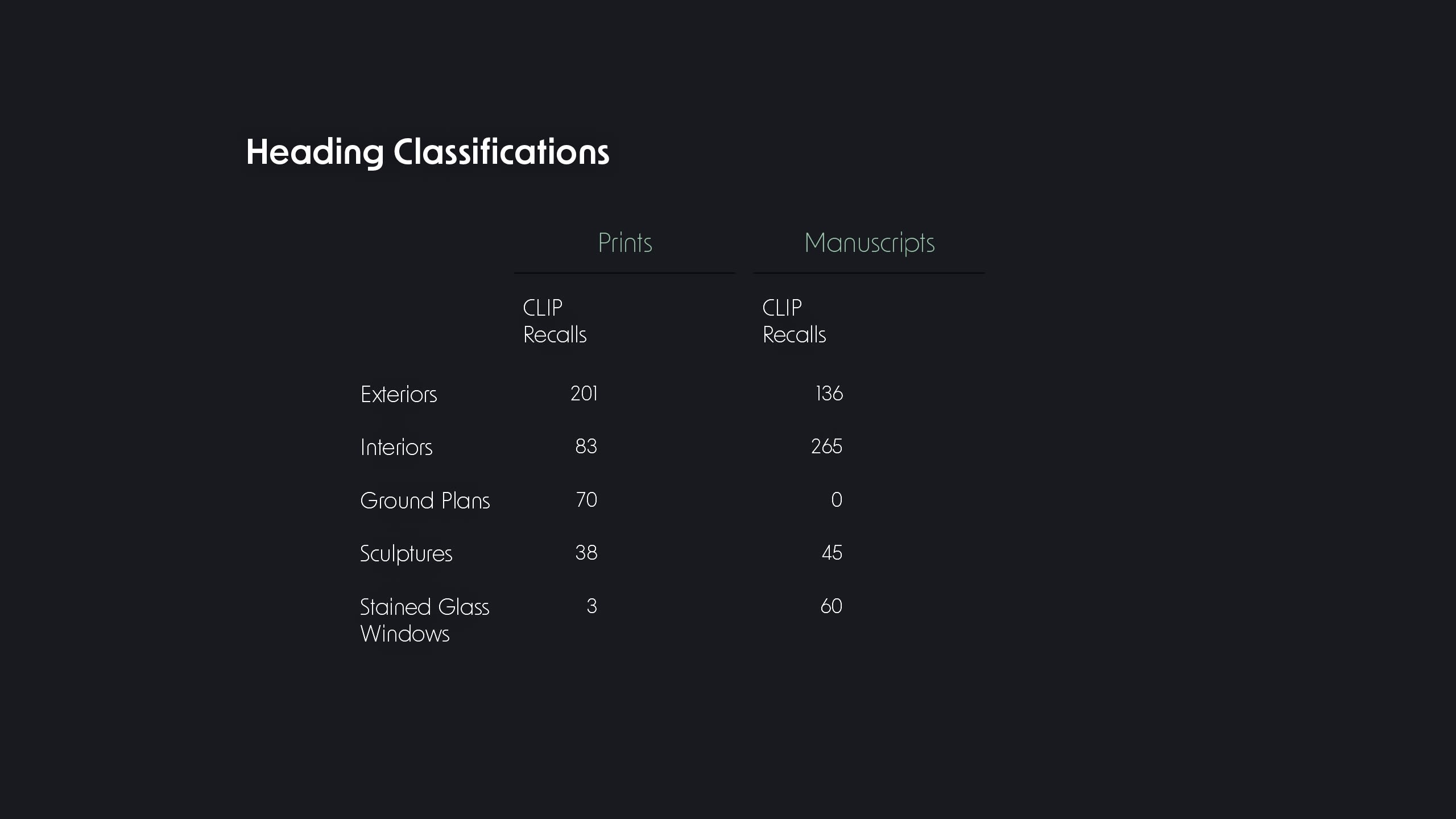
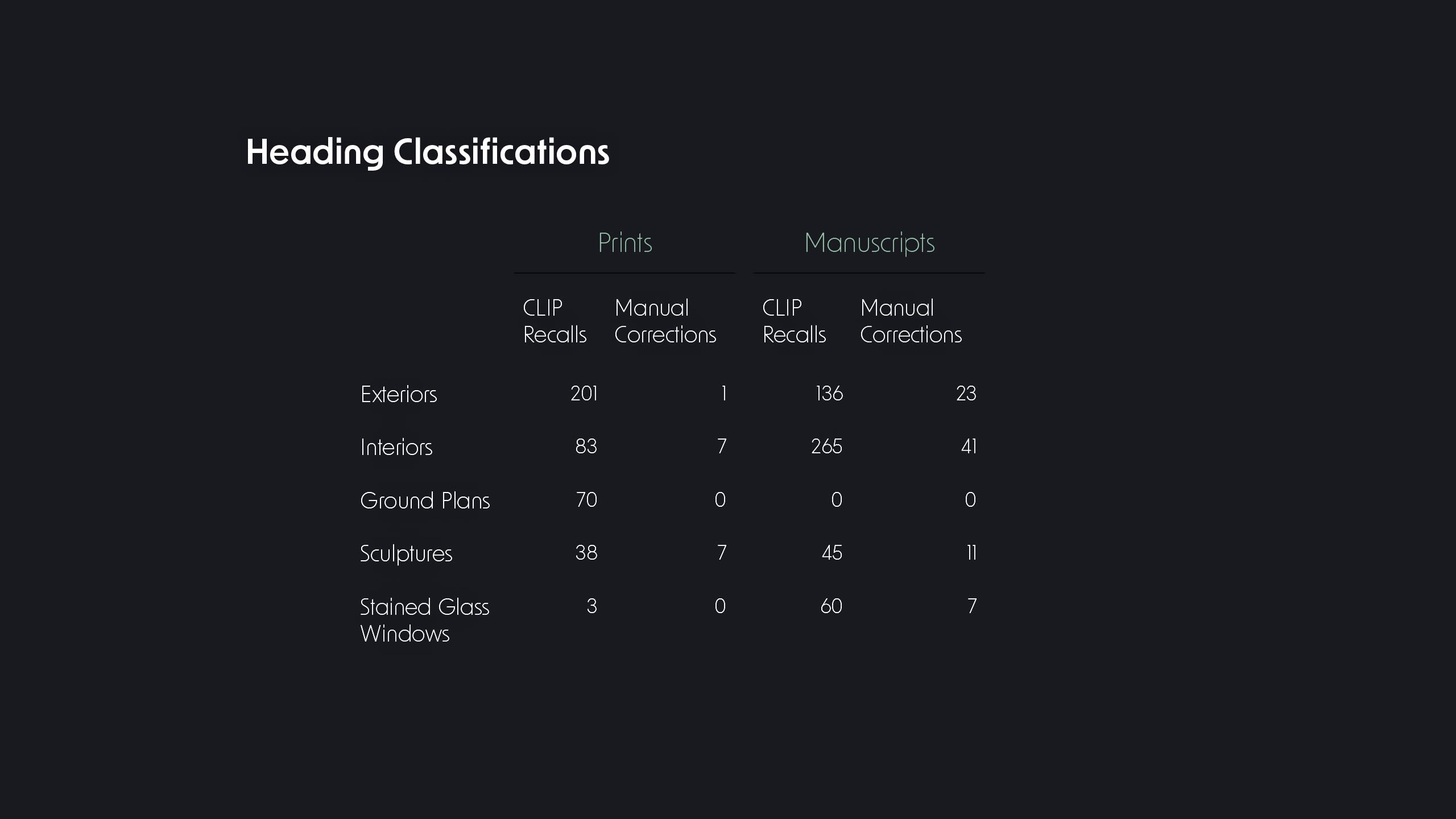
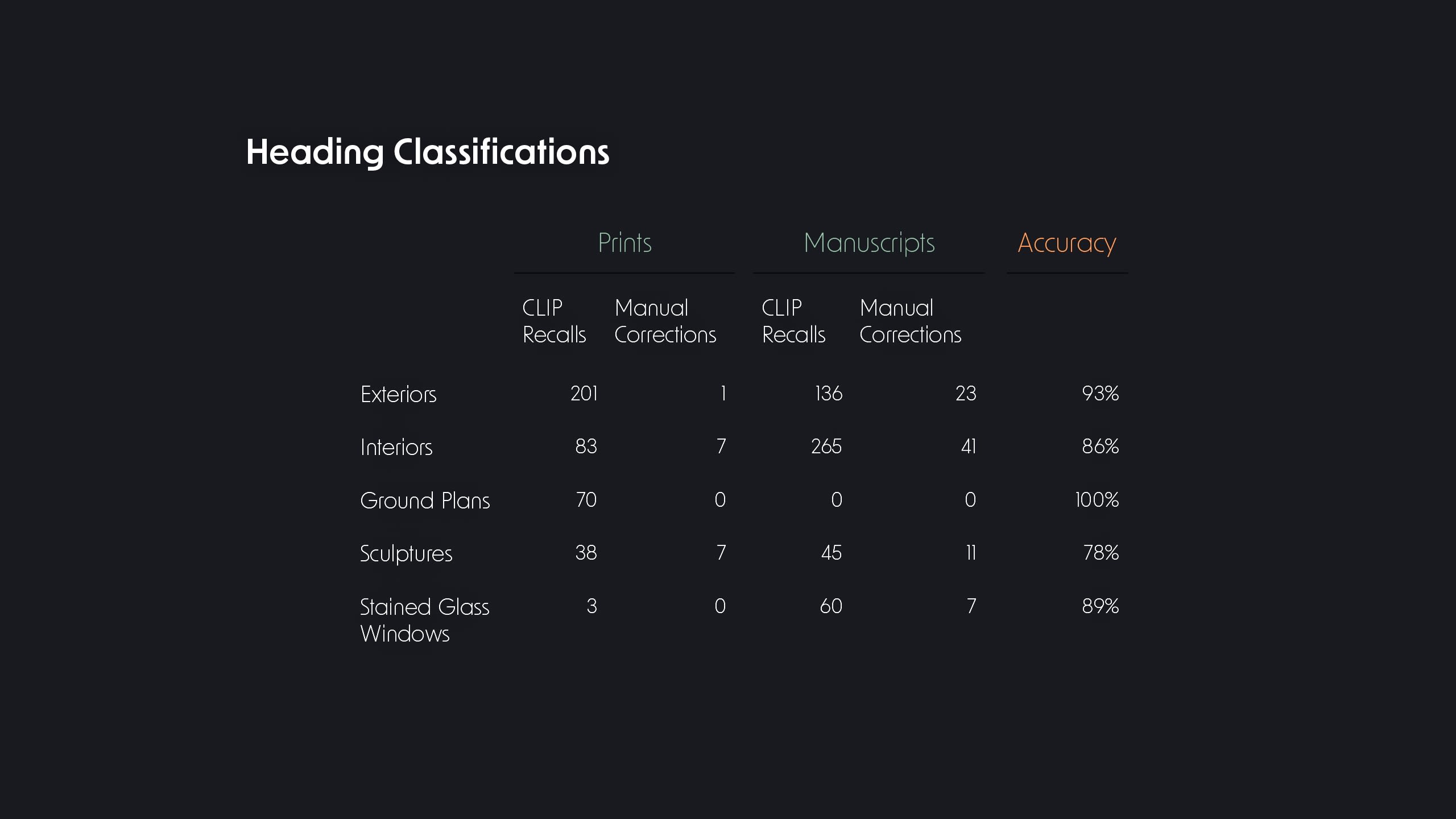
From Headings to Categorisation of Architectural features
With a high level of accurate results, we leveraged the heading classifications to conduct more granular queries to analyse our dataset with greater precision. This approach significantly enhanced our ability to explore the capabilities of image recognition.
To assess how effectively CLIP could identify and distinguish architectural features such as facades, naves, spires, altars, chapels, and vaults, we fine-tuned the model and implemented OWL-ViT to enhance its object detection capabilities. This allowed us to evaluate both how CLIP could classify these architectural terms and how accurately it could localise them within images using bounding boxes.
As CLIP, fine-tuned with OWL-ViT, "scans" the image, it attempts to detect features that correspond to the provided textual label. Once a relevant feature is found, the model delineates its location.
Bounding boxes visually indicate where the model identifies a feature and includes a confidence score, reflecting the likelihood of a correct match. These boxes are essential for assessing the model's accuracy in detecting and classifying architectural features.
By combining CLIP's robust text-image matching abilities with precise localisation, we aimed to assess the model's ability to handle more specialised textual queries tied to the intricate features of cathedral exteriors and interiors for advanced visual analysis.
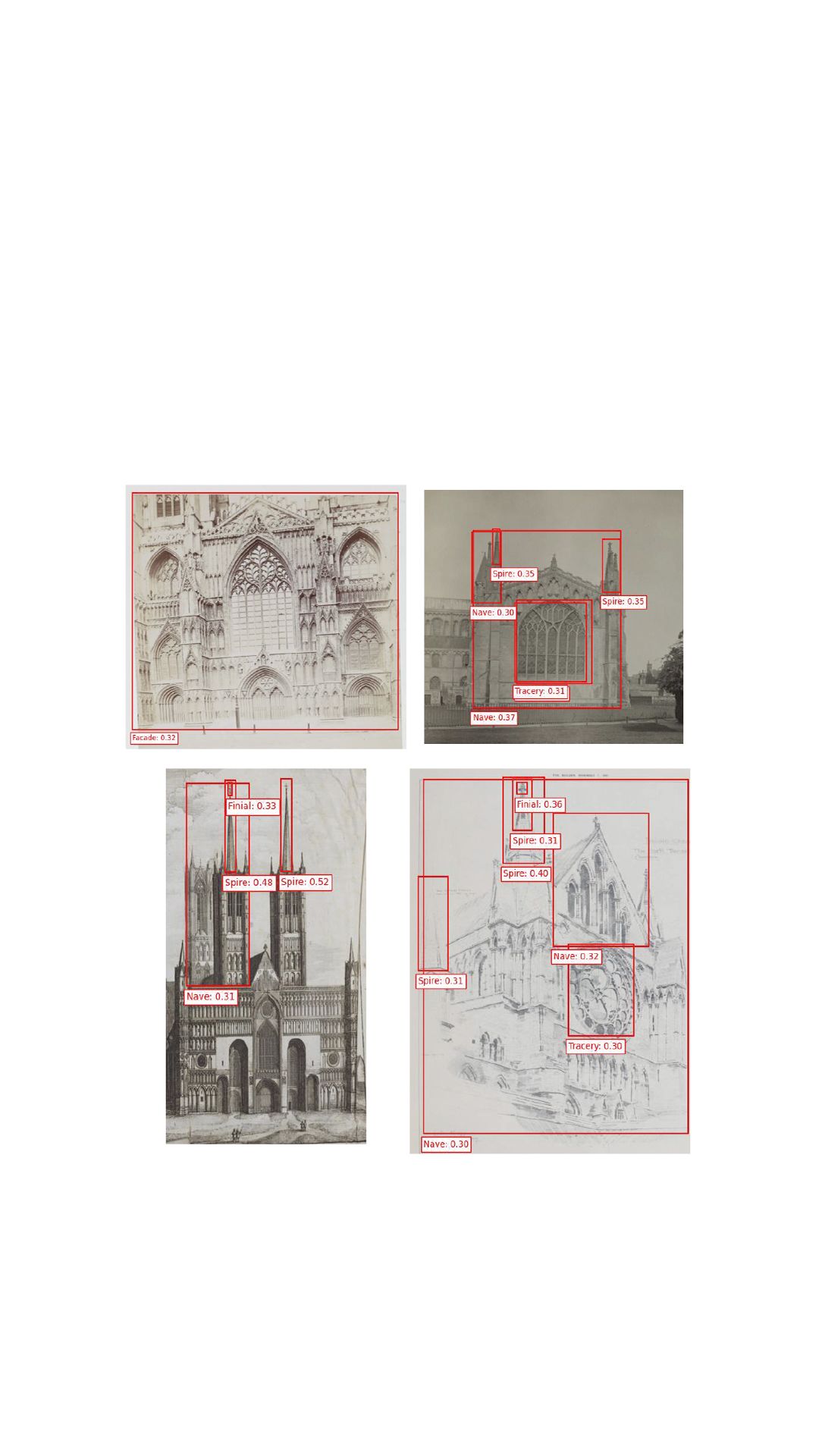
The analysis of categories within the interior classification revealed distinct challenges. While the model successfully identified numerous features such as altars, columns, chapels, and lecterns, it noticeably missed other key architectural elements , including stained-glass windows, vaults, and arches. These gaps in detection became evident during the analysis. To address this limitation, we conducted additional tests by lowering the confidence threshold, enabling the model to capture a broader range of potential matches for interior features. This adjustment allowed for a more comprehensive exploration of the dataset, ensuring fewer architectural details were missed.
Confidence threshold
To improve the model's ability to detect intricate features, such as stained-glass windows, we lowered the confidence threshold. This adjustment allowed CLIP to capture a wider range of text-image associations, increasing recall and ensuring that vital details were not overlooked.
Enhance Recall for Comprehensive Analysis: In situations where a model might miss important elements because its confidence threshold is set too high, lowering the threshold helps to catch a greater number of relevant items. For projects like studying medieval architecture, it is crucial to recognise as many architectural features as possible, even if some identifications require manual verification.
Adaptation to Non-Ideal Data: Historical prints and photographs, particularly non-born-digital assets, often have lower clarity and more variability compared to modern, high-resolution digital images. By reducing the confidence threshold, the model can accommodate these less-than-ideal conditions, ensuring it doesn’t overlook valuable details.
Exploratory Research: For research-driven projects, a higher recall rate allows for more exploratory analysis. Even though it may come at the cost of reduced precision (potentially more false positives), researchers can manually filter or further process the data to extract meaningful insights. This trade-off can be particularly beneficial when aiming to catalogue comprehensive visual collections.
Enabling Further Fine-Tuning: Running a model with a lower threshold provides a larger dataset of matches that can be analysed for patterns. These findings can guide subsequent model improvements or manual curation, helping to refine the AI's performance over time.
Lowering the confidence threshold allowed the model to validate a broader range of text-image associations, significantly enhancing its ability to identify intricate details like stained-glass windows within our non-born-digital dataset. This adjustment vastly improved the recall rate, helping the model to uncover features that might have otherwise been overlooked due to the variability and complexity of the library's historical collection.
While reducing the confidence score does involve a trade-off—capturing more matches at the cost of precision—it proved highly effective for our goals. The increase in accurate results for stained-glass windows, a defining element of medieval cathedral architecture, demonstrates the value of this approach. For researchers focusing on such specific features, the ability to filter and locate relevant images with precision is an invaluable tool, opening up new pathways for detailed analysis and study.
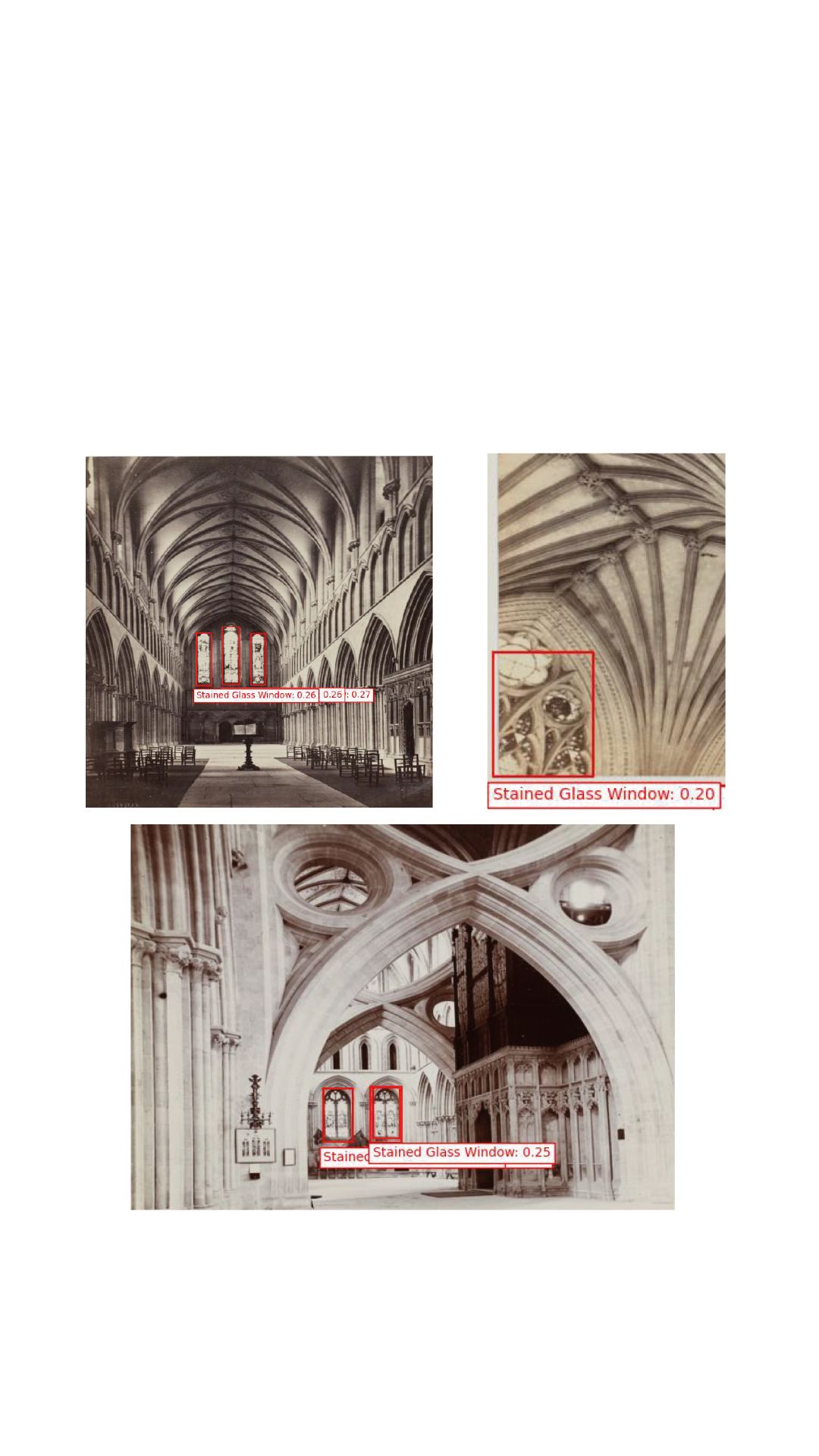
After improving the model's recognition of stained-glass windows, we applied the same approach to other architectural features, such as vaults and arches. Building on this success, we expanded our testing to see if CLIP's Natural Language Processing capabilities could detect the stylistic variations and specific types of vaults and arches, further enhancing the depth of our analysis.
Instead of relying solely on single-term queries, we crafted detailed full-text descriptions to guide the model in identifying nuanced differences. This not only improved the precision of stylistic identification but also enhances the accessibility of the image content.
For example lierne vault: "A complex ribbed vault featuring additional, shorter ribs, called liernes, that connect the main ribs without reaching the central point, creating intricate star-like or web-like patterns." This description emphasises the distinctive feature of a lierne vault—its use of extra ribs (liernes) that form decorative, intricate patterns, setting it apart from simpler ribbed vaults.
Or quadripartite vault: "A type of ribbed vault divided into four distinct sections or bays by two diagonal ribs that intersect at the centre, forming a cross-shaped pattern." This description highlights the main features of a quadripartite vault, emphasising its four-part division, the use of intersecting ribs, and the resulting cross shape.
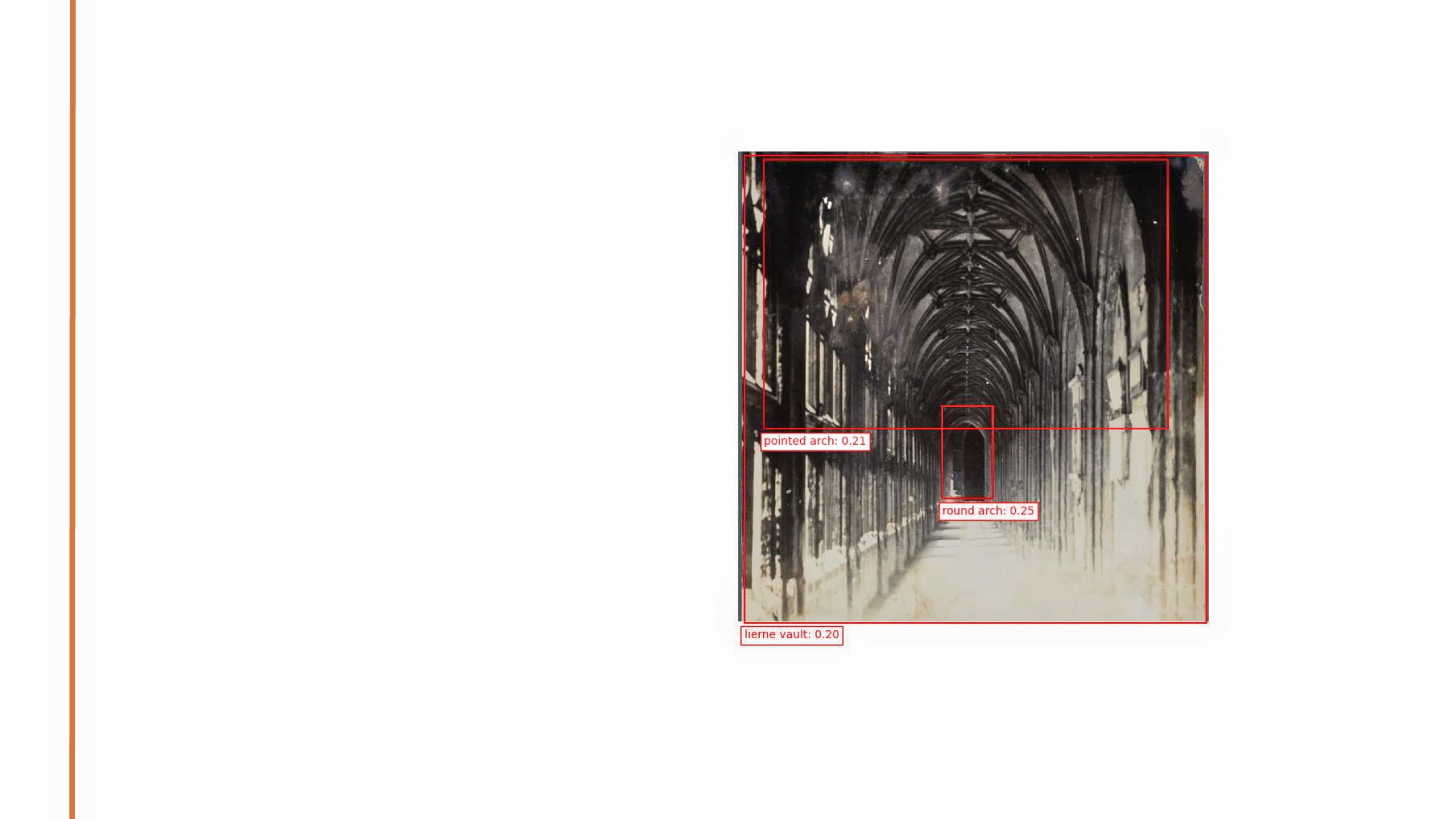
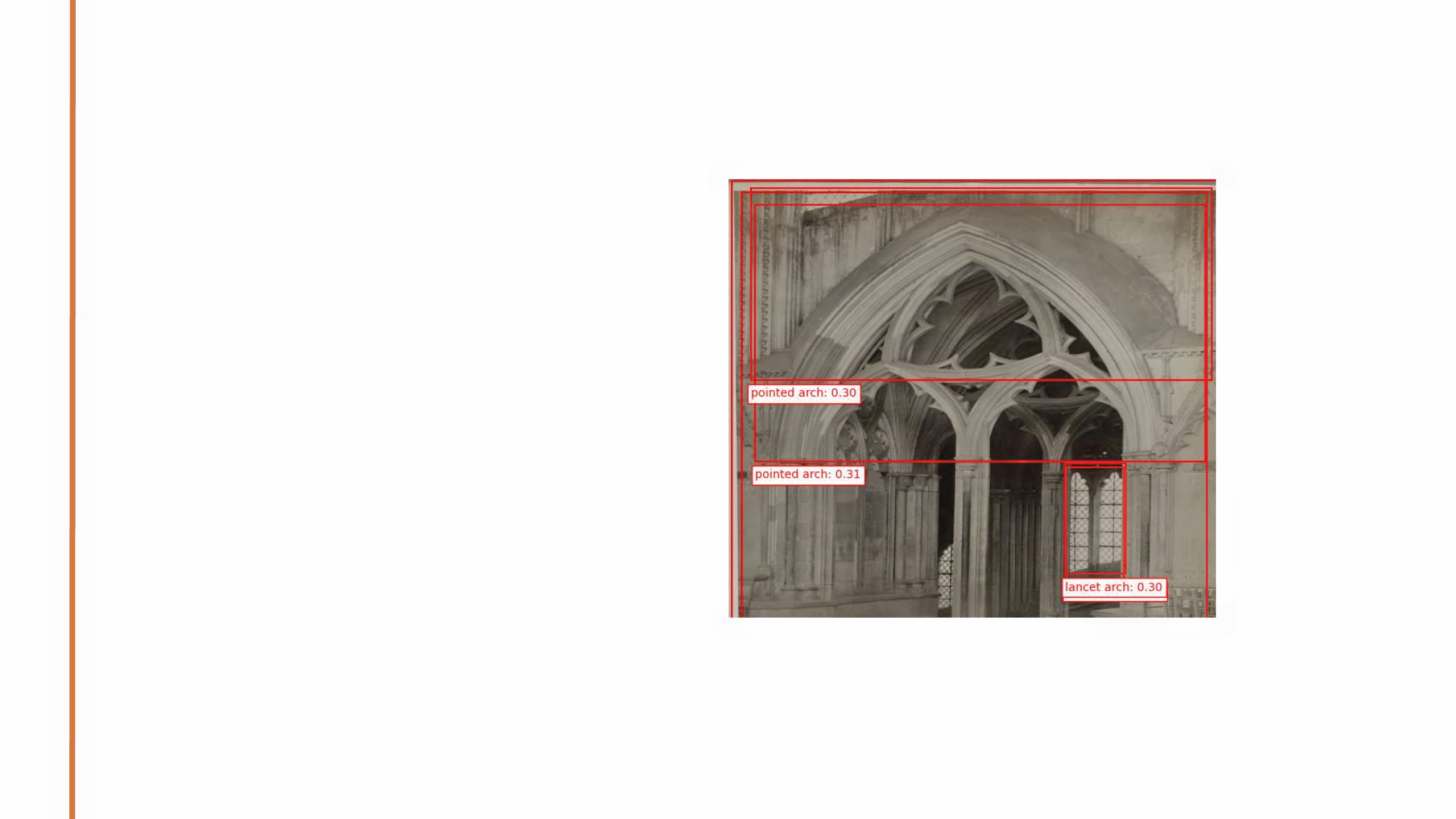
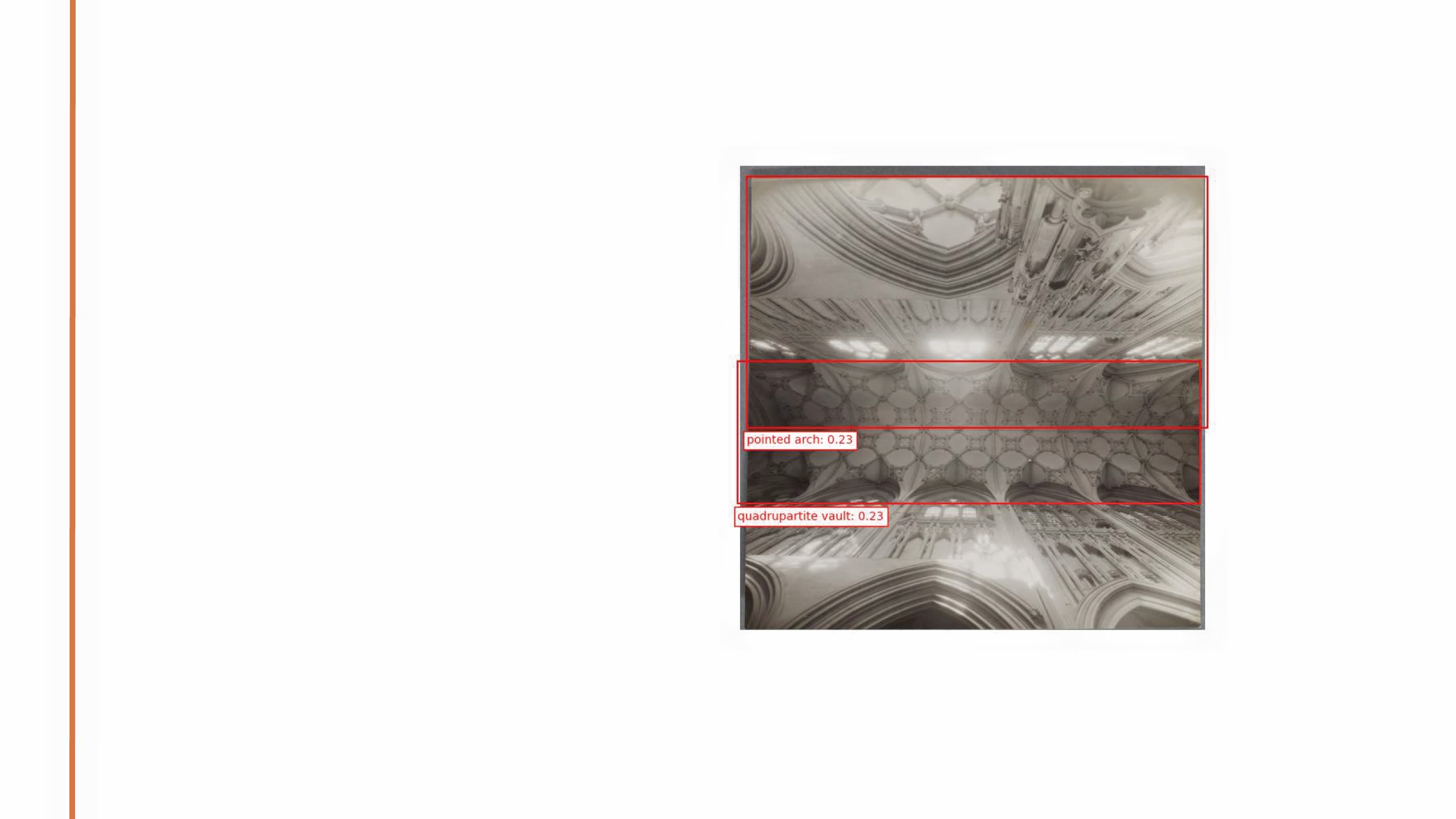
Challenges and Insights in Text Extraction: OCR vs. Caption Detection
Ground plans were an interesting subset to extract because, when combined with prints, they formed a strong cluster of image and text-based records that were ideal for testing caption detection and OCR.
While OCR faced limitations due to the poor quality of some historical materials—such as faded ink, complex fonts, and handwriting— the fine-tuned caption detection model developed by Irene Testini, proved far more effective. This method successfully identified and located textual information, even in challenging contexts where OCR struggled.
Given its ability to overcome OCR’s shortcomings, caption detection could be a critical tool in cataloguing processes, offering a reliable way to enhance the catalogue's accessibility by providing audiences with clearer indications of the textual content within images that might otherwise be overlooked.
Building on Cathedrals is a ground-breaking research project that merges the timeless beauty of medieval architecture with the transformative power of artificial intelligence. By harnessing cutting-edge AI technologies such as CLIP OWL-ViT, and caption detection, this project has not only preserved England's ecclesiastical heritage but also redefined how we study and interact with it. Through advanced image recognition and textual analysis, the project has illuminated intricate architectural features previously hidden within centuries-old records, offering an unprecedented level of detail and insight into medieval cathedral designs.
The project’s success lies in its ability to enhance accessibility to historical materials, allowing academics, cultural heritage conservators, architects, researchers, scholars, and enthusiasts to engage with these architectural wonders like never before. Over 1,400 AI-enhanced tags have been added, enriching the search and classification of thousands of images, making it easier to explore the richness of medieval cathedrals. The AI-driven classification has fostered collaboration among experts, deepening the library's role as a dynamic resource for cultural heritage and academic research.
Building on Cathedrals exemplifies the vast potential of digital humanities, demonstrating how AI can push the boundaries of traditional research, while also preserving fragile historical materials for future generations. As visitors explore the AI-enhanced collection, they are invited to uncover new layers of meaning, challenge conventional perspectives, and contribute to a future of deeper, more nuanced exploration of medieval cathedrals.
This project and exhibition is not just a digital showcase; it is a transformative milestone in the intersection of technology, history, and the preservation of cultural heritage, setting the stage for an exciting era of discovery and scholarship at Lambeth Palace Library.
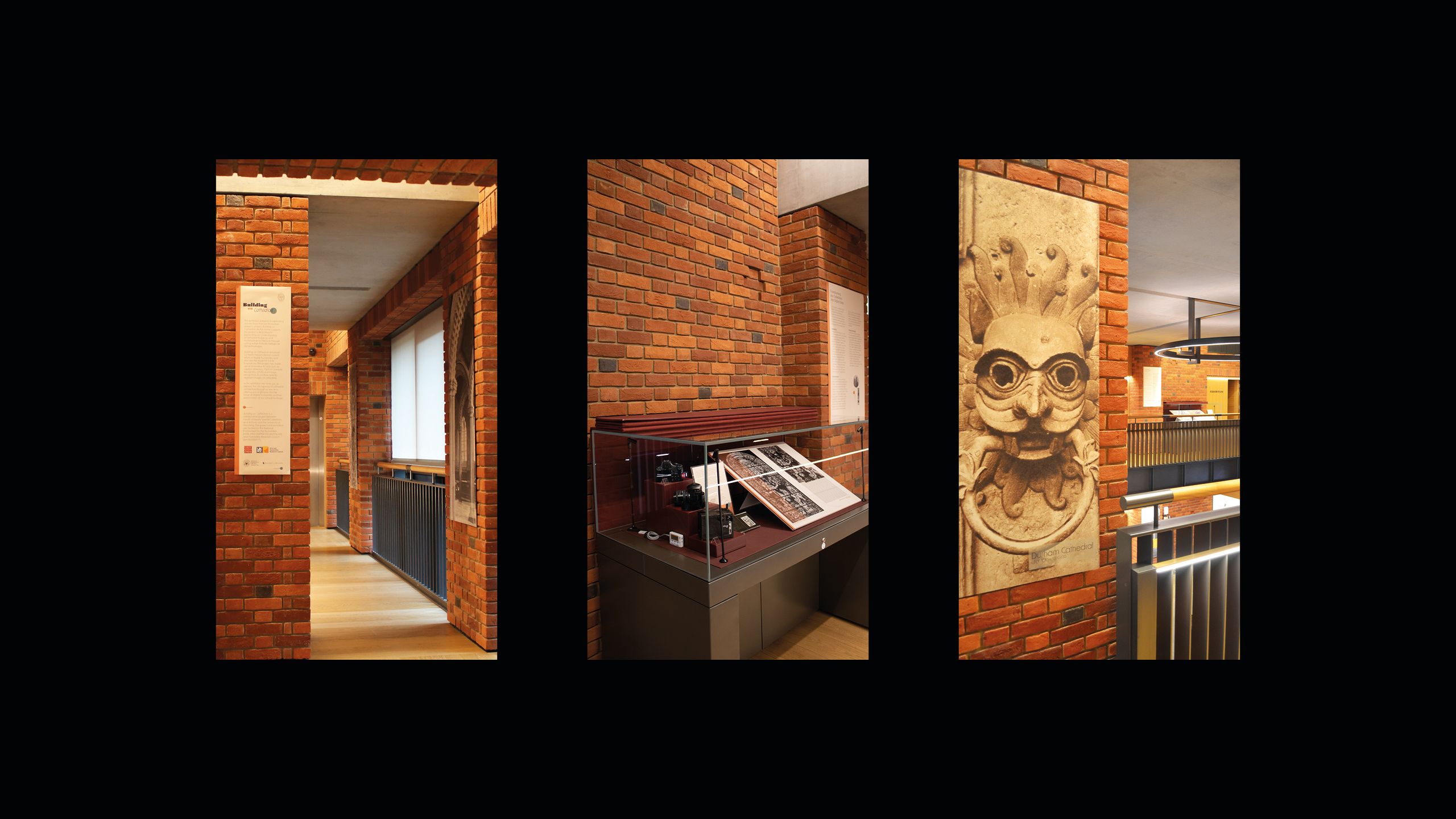
Building on Cathedrals Exhibition
Lambeth Palace Library
2 September - 20 December 2024
Find more information and to download the resources and tools developed for Finding A Place please use the following link: findingaplace.org.uk.
If you are interested in finding our more about the code visit: https://github.com/irene-crepax/FindingAPlace
Project Research, Exhibition Curation, Graphic Design and Digital Exhibition created by Camille Koutoulakis